تاريخ الرياضيات
الاعداد و نظريتها
تاريخ التحليل
تار يخ الجبر
الهندسة و التبلوجي
الرياضيات في الحضارات المختلفة
العربية
اليونانية
البابلية
الصينية
المايا
المصرية
الهندية
الرياضيات المتقطعة
المنطق
اسس الرياضيات
فلسفة الرياضيات
مواضيع عامة في المنطق
الجبر
الجبر الخطي
الجبر المجرد
الجبر البولياني
مواضيع عامة في الجبر
الضبابية
نظرية المجموعات
نظرية الزمر
نظرية الحلقات والحقول
نظرية الاعداد
نظرية الفئات
حساب المتجهات
المتتاليات-المتسلسلات
المصفوفات و نظريتها
المثلثات
الهندسة
الهندسة المستوية
الهندسة غير المستوية
مواضيع عامة في الهندسة
التفاضل و التكامل
المعادلات التفاضلية و التكاملية
معادلات تفاضلية
معادلات تكاملية
مواضيع عامة في المعادلات
التحليل
التحليل العددي
التحليل العقدي
التحليل الدالي
مواضيع عامة في التحليل
التحليل الحقيقي
التبلوجيا
نظرية الالعاب
الاحتمالات و الاحصاء
نظرية التحكم
بحوث العمليات
نظرية الكم
الشفرات
الرياضيات التطبيقية
نظريات ومبرهنات
علماء الرياضيات
500AD
500-1499
1000to1499
1500to1599
1600to1649
1650to1699
1700to1749
1750to1779
1780to1799
1800to1819
1820to1829
1830to1839
1840to1849
1850to1859
1860to1864
1865to1869
1870to1874
1875to1879
1880to1884
1885to1889
1890to1894
1895to1899
1900to1904
1905to1909
1910to1914
1915to1919
1920to1924
1925to1929
1930to1939
1940to the present
علماء الرياضيات
الرياضيات في العلوم الاخرى
بحوث و اطاريح جامعية
هل تعلم
طرائق التدريس
الرياضيات العامة
نظرية البيان
Normal Distribution
المؤلف:
Beyer, W. H.
المصدر:
CRC Standard Mathematical Tables, 28th ed. Boca Raton, FL: CRC Press
الجزء والصفحة:
...
10-4-2021
3989
Normal Distribution
A normal distribution in a variate with mean
and variance
is a statistic distribution with probability density function
![]() |
(1) |
on the domain . While statisticians and mathematicians uniformly use the term "normal distribution" for this distribution, physicists sometimes call it a Gaussian distribution and, because of its curved flaring shape, social scientists refer to it as the "bell curve." Feller (1968) uses the symbol
for
in the above equation, but then switches to
in Feller (1971).
de Moivre developed the normal distribution as an approximation to the binomial distribution, and it was subsequently used by Laplace in 1783 to study measurement errors and by Gauss in 1809 in the analysis of astronomical data (Havil 2003, p. 157).
The normal distribution is implemented in the Wolfram Language as NormalDistribution[mu, sigma].
The so-called "standard normal distribution" is given by taking and
in a general normal distribution. An arbitrary normal distribution can be converted to a standard normal distribution by changing variables to
, so
, yielding
![]() |
(2) |
The Fisher-Behrens problem is the determination of a test for the equality of means for two normal distributions with different variances.
The normal distribution function gives the probability that a standard normal variate assumes a value in the interval
,
![]() |
![]() |
![]() |
(3) |
![]() |
![]() |
![]() |
(4) |
where erf is a function sometimes called the error function. Neither nor erf can be expressed in terms of finite additions, subtractions, multiplications, and root extractions, and so both must be either computed numerically or otherwise approximated.
The normal distribution is the limiting case of a discrete binomial distribution as the sample size
becomes large, in which case
is normal with mean and variance
![]() |
![]() |
![]() |
(5) |
![]() |
![]() |
![]() |
(6) |
with .
The distribution is properly normalized since
![]() |
(7) |
The cumulative distribution function, which gives the probability that a variate will assume a value , is then the integral of the normal distribution,
![]() |
![]() |
![]() |
(8) |
![]() |
![]() |
![]() |
(9) |
![]() |
![]() |
![]() |
(10) |
where erf is the so-called error function.
Normal distributions have many convenient properties, so random variates with unknown distributions are often assumed to be normal, especially in physics and astronomy. Although this can be a dangerous assumption, it is often a good approximation due to a surprising result known as the central limit theorem. This theorem states that the mean of any set of variates with any distribution having a finite mean and variance tends to the normal distribution. Many common attributes such as test scores, height, etc., follow roughly normal distributions, with few members at the high and low ends and many in the middle.
Because they occur so frequently, there is an unfortunate tendency to invoke normal distributions in situations where they may not be applicable. As Lippmann stated, "Everybody believes in the exponential law of errors: the experimenters, because they think it can be proved by mathematics; and the mathematicians, because they believe it has been established by observation" (Whittaker and Robinson 1967, p. 179).
Among the amazing properties of the normal distribution are that the normal sum distribution and normal difference distribution obtained by respectively adding and subtracting variates and
from two independent normal distributions with arbitrary means and variances are also normal! The normal ratio distribution obtained from
has a Cauchy distribution.
Using the k-statistic formalism, the unbiased estimator for the variance of a normal distribution is given by
![]() |
(11) |
where
![]() |
(12) |
so
![]() |
(13) |
The characteristic function for the normal distribution is
![]() |
(14) |
and the moment-generating function is
![]() |
![]() |
![]() |
(15) |
![]() |
![]() |
![]() |
(16) |
![]() |
![]() |
![]() |
(17) |
so
![]() |
![]() |
![]() |
(18) |
![]() |
![]() |
![]() |
(19) |
and
![]() |
![]() |
![]() |
(20) |
![]() |
![]() |
![]() |
(21) |
These can also be computed using
![]() |
![]() |
![]() |
(22) |
![]() |
![]() |
![]() |
(23) |
![]() |
![]() |
![]() |
(24) |
yielding, as before,
![]() |
![]() |
![]() |
(25) |
![]() |
![]() |
![]() |
(26) |
The raw moments can also be computed directly by computing the raw moments ,
![]() |
(27) |
(Papoulis 1984, pp. 147-148). Now let
![]() |
![]() |
![]() |
(28) |
![]() |
![]() |
![]() |
(29) |
![]() |
![]() |
![]() |
(30) |
giving the raw moments in terms of Gaussian integrals,
![]() |
(31) |
Evaluating these integrals gives
![]() |
![]() |
![]() |
(32) |
![]() |
![]() |
![]() |
(33) |
![]() |
![]() |
![]() |
(34) |
![]() |
![]() |
![]() |
(35) |
![]() |
![]() |
![]() |
(36) |
Now find the central moments,
![]() |
![]() |
![]() |
(37) |
![]() |
![]() |
![]() |
(38) |
![]() |
![]() |
![]() |
(39) |
![]() |
![]() |
![]() |
(40) |
The variance, skewness, and kurtosis excess are given by
![]() |
![]() |
![]() |
(41) |
![]() |
![]() |
![]() |
(42) |
![]() |
![]() |
![]() |
(43) |
The cumulant-generating function for a normal distribution is
![]() |
![]() |
![]() |
(44) |
![]() |
![]() |
![]() |
(45) |
so
![]() |
![]() |
![]() |
(46) |
![]() |
![]() |
![]() |
(47) |
![]() |
![]() |
![]() |
(48) |
For normal variates, for
, so the variance of k-statistic
is
![]() |
![]() |
![]() |
(49) |
![]() |
![]() |
![]() |
(50) |
Also,
![]() |
![]() |
![]() |
(51) |
![]() |
![]() |
![]() |
(52) |
![]() |
![]() |
![]() |
(53) |
where
![]() |
![]() |
![]() |
(54) |
![]() |
![]() |
![]() |
(55) |
The variance of the sample variance for a general distribution is given by
![]() |
(56) |
which simplifies in the case of a normal distribution to
![]() |
(57) |
(Kenney and Keeping 1951, p. 164).
If is a normal distribution, then
![]() |
(58) |
so variates with a normal distribution can be generated from variates
having a uniform distribution in (0,1) via
![]() |
(59) |
However, a simpler way to obtain numbers with a normal distribution is to use the Box-Muller transformation.
The differential equation having a normal distribution as its solution is
![]() |
(60) |
since
![]() |
(61) |
![]() |
(62) |
![]() |
(63) |
This equation has been generalized to yield more complicated distributions which are named using the so-called Pearson system.
The normal distribution is also a special case of the chi-squared distribution, since making the substitution
![]() |
(64) |
gives
![]() |
![]() |
![]() |
(65) |
![]() |
![]() |
![]() |
(66) |
Now, the real line is mapped onto the half-infinite interval
by this transformation, so an extra factor of 2 must be added to
, transforming
into
![]() |
![]() |
![]() |
(67) |
![]() |
![]() |
![]() |
(68) |
(Kenney and Keeping 1951, p. 98), where use has been made of the identity . As promised, (68) is a chi-squared distribution in
with
(and also a gamma distribution with
and
).
REFERENCES:
Beyer, W. H. CRC Standard Mathematical Tables, 28th ed. Boca Raton, FL: CRC Press, pp. 533-534, 1987.
Feller, W. An Introduction to Probability Theory and Its Applications, Vol. 1, 3rd ed. New York: Wiley, 1968.
Feller, W. An Introduction to Probability Theory and Its Applications, Vol. 2, 3rd ed. New York: Wiley, p. 45, 1971.
Havil, J. Gamma: Exploring Euler's Constant. Princeton, NJ: Princeton University Press, p. 157, 2003.
Kenney, J. F. and Keeping, E. S. Mathematics of Statistics, Pt. 2, 2nd ed. Princeton, NJ: Van Nostrand, 1951.
Kraitchik, M. "The Error Curve." §6.4 in Mathematical Recreations. New York: W. W. Norton, pp. 121-123, 1942.
Papoulis, A. Probability, Random Variables, and Stochastic Processes, 2nd ed. New York: McGraw-Hill, pp. 100-101, 1984.
Patel, J. K. and Read, C. B. Handbook of the Normal Distribution. New York: Dekker, 1982.
Spiegel, M. R. Theory and Problems of Probability and Statistics. New York: McGraw-Hill, pp. 109-111, 1992.
Steinhaus, H. Mathematical Snapshots, 3rd ed. New York: Dover, pp. 285-290, 1999.
Whittaker, E. T. and Robinson, G. "Normal Frequency Distribution." Ch. 8 in The Calculus of Observations: A Treatise on Numerical Mathematics, 4th ed. New York: Dover, pp. 164-208, 1967.
الاكثر قراءة في الاحتمالات و الاحصاء
اخر الاخبار
اخبار العتبة العباسية المقدسة
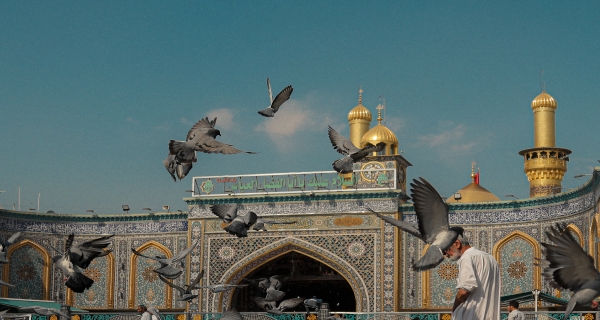
الآخبار الصحية
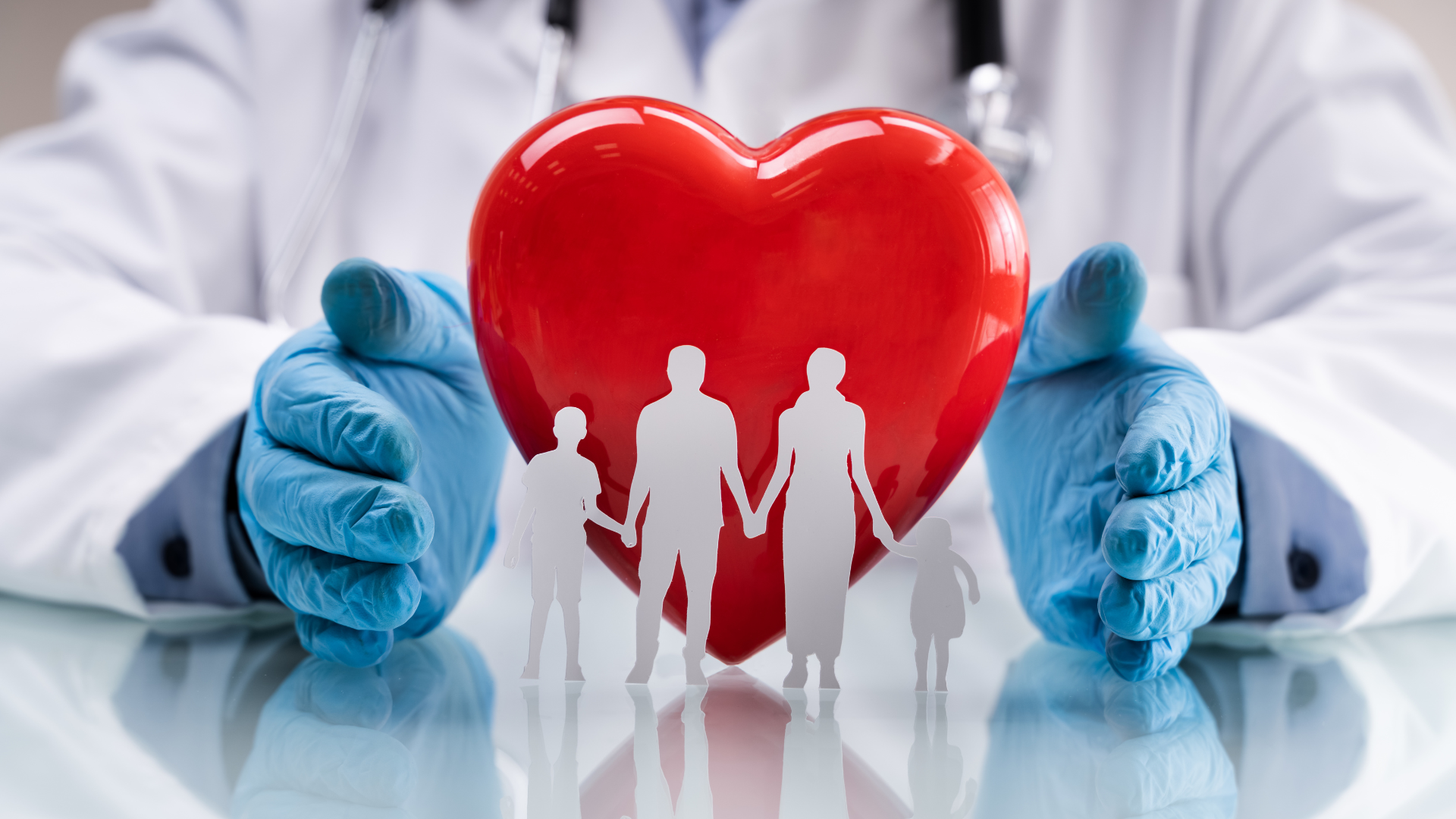